Elie J. Christoforides BS; Duncan Mulroy BS; Samuel Oswald BS; Brandon D. Rust BS; Rohit Muralidhar BS; Robin J. Jacobs PhD, MSW, MS, MPH
Nova Southeastern University Dr. Kiran C. Patel College of Osteopathic Medicine
DOI: 10.70709/2025-ZP987
Abstract
Background
Fracture types, resulting from both acute and chronic conditions, vary widely, including closed, open, stress, and pathological fractures. Trauma, falls, and sports injuries are leading causes, particularly among younger males, while factors like age, gender, and bone density significantly influence fracture susceptibility. Artificial intelligence (AI), through machine learning (ML) and convolutional neural networks (CNN), has emerged as a powerful tool in fracture detection, improving diagnostic accuracy and reducing errors in interpretation. Radiological AI algorithms are increasingly used in clinical settings, offering reliable diagnostic performance on par with trained clinicians. .
Purpose/Hypothesis
This review examines the role of AI in fracture detection, focusing on its accuracy compared to physician diagnoses and its potential to enhance clinical outcomes across different anatomical regions of the appendicular skeleton.
Study/Design
The exploration includes the AI’s role in enhancing clinical care, its comparative accuracy with trained clinicians, and its potential in minimizing diagnostic disparities during trauma-related scenarios.
Methods
A systematic search was conducted across multiple databases including Cochrane Central Register of Controlled Trials, Embase, OVID Medline, PubMed, and Web of Science, following the PRISMA guidelines for studies published in the English language between January 1, 2018 to December 31, 2022. Inclusion criteria targeted studies utilizing artificial intelligence modalities, radiographic images of pediatric or adult fractures, and the participation of orthopedic surgeons or radiologists for comparison of diagnostic accuracy with AI.
Results
A total of 754 articles were screened, with 36 meeting inclusion criteria for this review. These studies focused on AI-based fracture detection compared directly with physician diagnoses, specifically for appendicular skeleton fractures. Among the included articles, 13 compared AI to radiologists, 3 to orthopedic surgeons, and 1 to ER physicians, with 19 involving unspecified or mixed specialties. AI models were categorized by type, with 12 using Convolutional Neural Networks (CNNs), 14 using Deep Convolutional Neural Networks (DCNNs), and 10 employing unspecified deep learning models. Regarding fracture locations, 5 studies focused on the distal radius, 4 on wrist bones, 2 each on ankle and humerus fractures, 4 on the scaphoid, 6 on the hip, and 3 on the femur, while 7 evaluated fractures across the appendicular skeleton. Most studies were retrospective cohort designs (n=35), with one prospective study included. A general population was examined in 28 studies, while 5 focused on pediatric fractures, and 1 excluded pediatric images. X-rays were the primary imaging modality in 35 studies, with 1 study using MRI and CT. Comparative results indicated that AI outperformed physicians in 7 studies, matched their performance in 10, and was outperformed by physicians in 1. Physician performance improved with AI assistance in 11 studies. Additionally, 8 studies compared AI to “ground-truth” diagnoses to assess sensitivity and specificity. This categorization highlights the range of AI model types, specialties, and comparison methods across the studies.
Conclusions
This review evaluates the application of AI in radiological fracture detection. While current AI algorithms demonstrate promising potential, our findings indicate a need for further improvement in predictive accuracy before broad clinical implementation. Multidisciplinary collaboration appears crucial for optimizing diagnostic outcomes and improving patient care.
Keywords: Fracture Detection, Artificial Intelligence, Machine Learning, Convolutional Neural Networks, Diagnostic Radiology, Appendicular Skeleton.
Introduction
Fractures
Fractures represent a common medical condition, either acute or chronic, encompassing various types such as closed and open fractures. Open fractures are defined by the bone protruding through the skin, while closed fractures do not exhibit this characteristic. Notably, 93% of limb fractures occur in isolation, with only 4% classified as open fractures, and the majority being closed fractures (1). Stress fractures, another type, arise from repetitive rather than sudden mechanical stress. Additionally, pathological fractures occur when underlying metabolic conditions or diseases weaken the bone’s structure, increasing the likelihood of fractures (2). Examining these categories is essential for developing effective fracture management and prevention strategies.
Fractures primarily result from multifactorial causes, with trauma and falls being the predominant etiologies (3). Sports activities, especially among young males, contribute significantly to fracture incidence (1). Several risk factors also influence fracture susceptibility, with age being a key determinant—older individuals often experience reduced bone density, increasing their fracture risk (3). Gender differences also exist, as postmenopausal women are at a higher risk due to hormonal changes, as highlighted by the World Health Organization (WHO) (4). Additionally, bone density, affected by genetics, lifestyle, smoking, exercise, and nutrition, is a crucial factor in fracture risk (4).
Anatomical terminology is essential in understanding fracture location and describing bone alignment. Terms like “proximal” and “distal” help indicate the position of bone fragments, with “proximal” referring to the part closest to the body’s center and “distal” referring to the furthest part. “Displacement” describes the degree of misalignment between bone fragments. Anatomical details are vital for fracture research, as certain sites are more vulnerable to injury. The distal radius and ulna are the most common fracture sites, with proximal humerus fractures following closely behind for upper extremity injuries (5). Among the elderly, foot fractures are frequent and correlate with significant declines in physical and social functioning (5). Proper alignment of bone fragments is critical for effective healing, minimizing complications, and improving patient outcomes.
Artificial Intelligence
Artificial intelligence (AI) is an emerging field that significantly impacts medicine. AI refers to algorithms that simulate human intelligence, encompassing subfields that have become particularly useful in radiology and disease identification from imaging. These subfields include machine learning (ML), neural networks, deep learning (DL), and convolutional neural networks (CNN) (6).
Machine learning (ML) involves an algorithm designed to learn from data inputs, becoming increasingly accurate over time. Neural networks, a subset of ML, utilize layers of algorithms that build upon one another, forming “deep neural networks” when layers become numerous, a characteristic of deep learning (6). Deep learning allows AI to perform perceptual tasks like image identification by processing large data sets quickly through advanced hardware (7). Convolutional neural networks (CNNs), a DL subset, have proven effective in medical imaging, as their design—modeled after the human visual cortex—enables them to generalize image perception across varied orientations while minimizing overfitting (7).
Artificial Intelligence in Diagnostic Radiology
Fracture detection through radiography is common in trauma-related scenarios across various clinical settings. Diagnostic errors, which may result in overlooked fractures, are frequent, with discrepancies of up to 24% between non-experts and board-certified physicians, particularly during evening and overnight shifts (5 PM to 3 AM) due to factors like fatigue [8]. Such discrepancies can lead to patient harm or delays in care.
AI is instrumental in fracture detection, reducing interpretation errors and speeding up diagnostics. Studies indicate that AI performs comparably to trained clinicians in identifying fractures, especially in regions such as the hand, wrist, forearm (8–11), pelvis, hip (12,13), knee (14), and spine (15). While AI-based detection holds promise, variations exist in defining clinician expertise and specialty training. This paper aims to present a comprehensive overview of AI’s efficacy in fracture detection relative to clinicians, examining the most accurate models and their limitations across upper and lower extremity anatomical regions.
Methods
Eligibility Criteria
To be eligible for inclusion, articles had to be original, peer-reviewed, written in English, and based on research studies conducted in the U.S. between January 1, 2018, and December 31, 2022. Additional criteria comprised the utilization of artificial intelligence modalities, such as machine learning, neural networks, and deep learning algorithms. The studies needed to involve radiographic images of pediatric or adult fractures and include study samples consisting of medical professionals, specifically orthopedic surgeons and radiologists, whose representation was evident in the findings.
Furthermore, the criteria encompassed the comparison of diagnostic ability and accuracy between these medical professionals and artificial intelligence as a significant finding in the study. The establishment of inclusion and exclusion criteria was undertaken to align with the goals of our scoping review. Excluded were background articles and presentations of studies solely predicting the efficacy of artificial intelligence or the accuracy of deep learning algorithms. Studies that either did not allude to physician usage or focused solely on artificial intelligence without directly comparing fracture detection accuracy were also excluded.
Our analysis was expanded to encompass studies that examined the appendicular skeleton of the upper and lower extremity, allowing for a broader exploration of anatomical significance and variability in fracture presentation encountered in clinical and hospital settings. Conversely, studies related to the axial skeleton were excluded to account for leading etiologies and risk factors such as age, gender, and bone density, which substantially influence the distal upper and lower extremities as the most common areas and thus provided more evidence for comparison.
We refined our sample by including studies with at least two themes: one addressing artificial intelligence modalities presenting data on localizing a pathological region and the other focusing on medical professionals’ judgment of the same radiographs. This approach ensured a direct comparison between the performance of the two, supported by statistical significance. For comprehensive representation of findings, medical physicians across any specialty were included, as long as there was a level of radiographic analysis involved in the study. Radiologists and orthopedic surgeons constituted a significant percentage of these participants.
Information Sources
All authors collaborated on developing a search strategy in consultation with the primary investigator. The foundational search strategy was formulated through an analysis of key terms and pertinent articles sourced from MEDLINE, EMBASE, and CINAHL. The exploration extended to databases such as EMBASE, Ovid Medicine, and Web of Sciences. All searches were conducted in October 2023, with the base search method tailored to each specific database.
The search process yielded a total of 1,054 citations. Eliminating duplicates resulted in the removal of 200 entries, with none flagged as ineligible by automation tools or for other reasons. This left a total of 754 studies for screening. The fourth and fifth authors meticulously reviewed each title and abstract, managed the abstract review process, and reached a consensus on articles deemed relevant for further consideration (n = 242 articles).
The first and second authors, acting as independent reviewers, scrutinized each full-text article (n = 294) using a screening form to validate inclusion criteria for each piece. Subsequently, the reviewers engaged in discussions for each full-text article under consideration for inclusion. In instances of discordance between the article and the inclusion criteria, the two reviewers presented the matter to a third independent reviewer. Deliberations ensued among all three reviewers until a unanimous agreement was reached. At this juncture, 197 articles were excluded for not meeting screening criteria. Specifically, 60 studies deviated from focusing on radiographs of the appendicular skeleton, 41 did not directly measure a physician’s analysis for comparison with that of the artificial intelligence modality, 27 were solely predictive assessments of their respective algorithm’s efficacy, 14 exhibited an incorrect outcome, 10 employed inappropriate study designs, and finally, 6 were in a foreign language. Consequently, 36 studies remained for further analysis. The screening and selection process was visually represented using the PRISMA flowchart in Figure 1.
Search Strategy
The research question was formulated using the Population, Concept, and Context (PCC) strategy, where “P” represents population, “C” denotes Artificial Intelligence (AI) in Orthopedics, and the second “C” signifies the use of AI in fracture detection, either in comparison with physician diagnosis or its supportive role in physicians’ diagnoses. Framed within these parameters, the guiding question aimed to explore the available literature evidence on the use of AI in contrast to the diagnostic accuracy of physicians in fracture detection. Data collection occurred in October 2023, with searches conducted in EMBASE, Ovid MEDLINE, and Web of Science. The choice of these databases was driven by the novelty of the subject and our specific inclusion criteria. Despite the abundance of articles on AI in Orthopedics, our focused subject matter necessitated the exploration of additional search databases. The search strategy required sources to be published in English and align with our predefined inclusion criteria.
The research was independently conducted by five medical student reviewers, each using controlled descriptors such as ‘Artificial Intelligence,’ ‘Deep Learning,’ ‘Machine Learning,’ ‘Diagnostic Detection,’ ‘Fracture,’ ‘Upper Extremity,’ ‘Lower Extremity,’ ‘Radiology,’ and ‘Orthopedic Injuries.’ These descriptors were combined in various ways to encompass all articles related to the subject matter. All searches utilized Boolean operators, particularly “AND,” to ensure the simultaneous occurrence of relevant subjects.
This review encompassed articles within the field of AI in Orthopedics concerning its role in aiding physicians in the detection and diagnosis of appendicular fractures or its performance in comparison to physicians. Inclusion criteria specified that articles must be published and in English, while exclusion criteria ruled out review studies, editorials, expert opinions, unpublished articles, and studies covering bone diseases beyond the scope of our interest. Specifically, articles solely focused on axial skeleton fractures were excluded. The exclusion did not consider the level of evidence, given the emerging nature of this field with a limited likelihood of finding articles with higher levels of evidence. A total of 754 articles were identified across the three databases, and the PRISMA method—Preferred Reporting Items for Systematic Reviews and Meta-Analyses (Tricco et al., 2018)—was adopted to systematically manage the inclusion process of the studies. A detailed search strategy is summarized in Table 1.
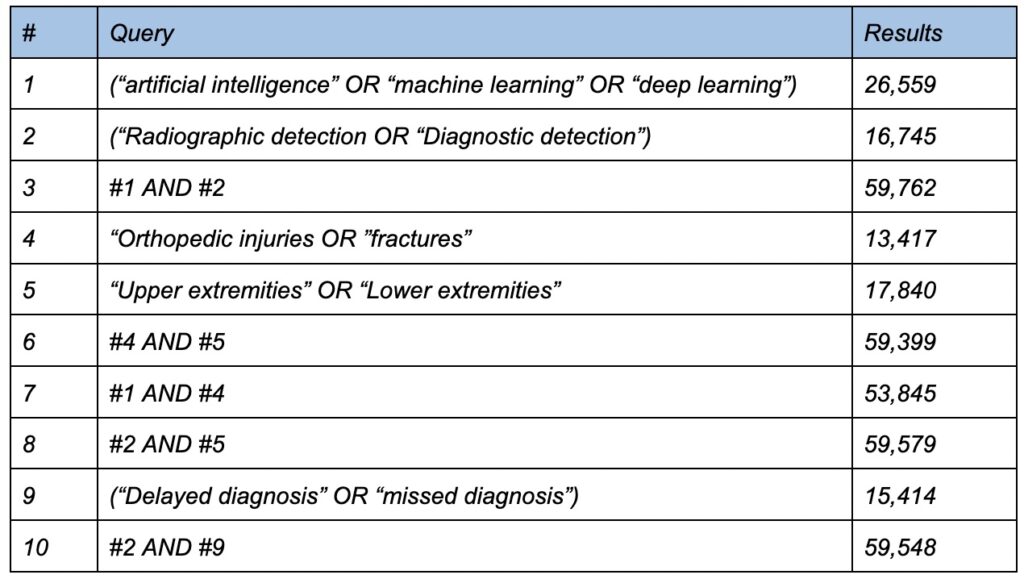
Selection of Sources of Evidence
A collaborative effort involving five reviewers, working in pairs, systematically assessed the titles, abstracts, and subsequent full-text publications of all studies identified through our searches for potential relevance. Any disagreements regarding study selection and data extraction were resolved through consensus within each pair, and further discussion with other reviewers was employed when necessary.
Data Charting Process:
In extracting pertinent information from our included sources of evidence, we employed a designed data charting form to ensure clarity and comprehensiveness. The selection of items for charting was driven by predefined research objectives, and inclusion and exclusion criteria were clearly outlined. Microsoft Excel served as our chosen software, and calibration among team members was achieved through a training session, which fostered uniformity in the charting process. The full process involved a team of three reviewers who worked collaboratively to extract data; if an inconsistency arose, then a team meeting was held to address and resolve it through a consensus-based approach. Data verification was carried out by selecting entries for cross-checking from a third reviewer, enhancing the accuracy and reliability of our findings. Throughout the iterative process, revisions to the charting form were documented, accompanied by clear rationales for each modification listed in the excel table.
Data Items
Data abstraction was based on a myriad of characteristics: having a gold standard comparison (e.g. radiologists, consensus panel of specialists, orthopedic surgeons), diverse dataset (e.g. range of radiograph anatomical locations, varying severities, pediatric and adult pathologies), large sample size, sensitivity and specificity analysis of the artificial intelligence modality (e.g. identity true positives [fractures] and true negatives [absence of fractures], clinical validation and real-world application (e.g. practical utility in healthcare workflow of the emergency room, operating room). By incorporating these characteristics, studies assessing the accuracy of AI in fracture detection can provide a comprehensive and reliable evaluation, helping clinicians and researchers better understand the strengths and limitations of AI in this critical medical domain.
Critical Appraisal
When choosing articles for a scoping review, it is crucial to assess them for quality, validity, and any biases, as these factors significantly impact the resulting review. The inclusion of articles with poor data or biases could compromise the integrity of our scoping review. To address this concern, we conducted a systematic review of all selected articles following the completion of tier-one screening.
In this process, we utilized the Joanna Briggs Institute Critical Appraisal Tools (JBI), a critical appraisal tool that provides guidance on utilizing article type-specific checklists. This ensures that any reviewer can accurately assess the quality of selected articles, maintaining consistency throughout the entire process. The JBI checklists consider research biases, overall congruence, and essential sections that contribute to an article’s quality.
The tools enabled us to categorize articles into three risk levels: high risk of bias (scores below 50%), moderate risk of bias (scores between 50% and 70%), and low risk of bias (scores above 70%). To enhance reliability, a minimum of two researchers conducted in-depth readings and blindly appraised all semifinalist articles using the relevant JBI appraisal tools. The appraisal process was thoroughly discussed among all five members of the research team, leading to the approval of final articles with scores above 70%.
Following critical appraisal, we made the decision to include 36 articles in the final scoping review, having narrowed down the tier-1 articles from an initial pool of 754.
Synthesis of Results
We categorized the studies based on their study designs (e.g., retrospective cohort studies, prospective studies) and presented a summary in tabular form. This table outlines the anatomical locations of different radiographic fractures, the subtype of artificial intelligence algorithm utilized, and the respective performance comparisons for each type of analysis.
Results
In this scoping review, 754 research articles were initially selected for evaluation. Of those 754 articles, 36 remained after the exclusion process. The flowchart above in Figure 1 maps out the different exclusion criteria and the number of articles that were removed after each round of selection. This scoping review focused on articles that included the use of physicians that were directly compared to an AI program. Although most of the articles included radiologists for comparison, there were a variety of other specialties that were also included. It is also important that the articles were separated based on the type of AI used since some deep learning models have more layers of data extraction which increase their predictive value. Another condition for an article to be included was the exclusive focus on fractures of the appendicular skeleton, however the majority of the articles focused more intently on fractures of a specific bone. Articles were further separated based on their study design and the population of focus. This was important because a small number of the articles focused exclusively on fractures of pediatric patients. Additionally, articles differed based on the type of imaging modality that was analyzed within the study. Finally, it is important to explain precisely how the AI models were compared to the physicians.
Physician Specialties
It is important to include multiple physician specialties in order to have a more precise comparison of the predictive accuracies of AI and the healthcare industry. Of the articles, 13 directly compared the AI’s fracture detection to radiologists exclusively (17-29). 3 articles focused on orthopedic surgeons (30-32) and 1 was centered around ER physicians (33). Unfortunately, the physicians used in some articles were either unspecified or included a mixture of different specialties [34-52].
Deep Learning Model Programs
Although each AI program is unique, most can be categorized as either a convolutional neural network (CNN) or a deep convolutional neural network (DCNN). The difference stems from the number of layers of data extraction used by the AI program. The more layers that are included in the deep learning model, the more accurate the predictive value will be. CNN’s include less than or equal to 2 layers of data extraction, while DCNN’s include more than 2 layers. Of the articles included in the review, 12 included CNNs (21, 24, 30-32, 34, 40, 42, 46, 48, 50, 51), 14 of them included DCNNs (22, 23, 26, 28, 29, 33, 35, 37, 38, 39, 41, 43, 45, 52), and 10 articles used an unspecified deep learning program (17-20, 25, 27, 36, 44, 47, 49).
Fracture Locations on the Appendicular skeleton
Although all the articles used in this review focus on fractures of the appendicular skeleton, it is important to inspect one specific type of fracture to formulate a more accurate benchmark of the AI’s predictive ability. Of the articles that focused on one fracture exclusively, 5 inspected the distal radius (17, 30, 31, 34, 42), 4 inspected the bones of the wrist (22, 25, 32, 33), 2 focused on fractures of the ankle bones (35, 51), 4 on the scaphoid (19, 24, 48, 51), 6 on the hips (28, 36-38, 50, 52), 2 on the humerus (21, 40), 1 on the bones of the chest (23), 1 on the bones of the foot (46), 3 on the femur (29, 32, 47), and 1 on the bones around the knee (49). It was also important to observe articles that include any fracture of the appendicular skeleton to inspect the AI’s ability to detect fractures spontaneously, as in a real world setting. There were 7 articles that included fractures of any bone in the appendicular skeleton (18, 20, 27, 41, 43-45).
Study Design
Most of the articles used in this review were Retrospective Cohort Studies (17, 18, 20-52). However, 1 prospective study was also included (19).
Population
To objectively inspect the AI’s ability to detect a wide variety of fractures, it would be prudent to inspect fractures in all patients. The majority of articles that were included were designed this way (17-24, 28-33, 35-42, 45, 46-52). Inversely, some articles only inspected pediatric fractures, which allowed the study to observe if the AI’s predictive ability could be applied to pediatric patients with a comparable level of accuracy. There were 5 studies that inspected pediatric images exclusively (25, 27, 34, 43, 45). One of the articles expressed their decision to remove pediatric images from the study to allow the deep learning program to operate with less contrasting variables (26).
Image Modality
Considering the fact that these articles functioned to inspect fracture detection, it is logical to expect radiographs to be the image modality of choice. For this reason, 35 of the 36 articles used X-rays exclusively (17-38, 40-52). Interestingly, one study thought to use a combination of MRI and CT scans to train their AI so they could take advantage of the increased detail found in 3D imaging (39).
Comparisons to the Physicians
Perhaps the most important aspect of these studies was the comparison of the fracture detection ability of the AI to those of practicing physicians. Of the articles used, 7 stated that the AI outperformed the physicians in focus (22, 29, 30, 42, 43, 50, 51). 10 articles stated that the performance of the AIs were comparable to the physicians’ performances (17, 21, 23, 24, 26, 31, 36, 40, 42, 52). 1 article indicated that the physicians could more accurately detect fractures compared to the AI (48). Another format that was implemented involved comparing the fracture detection of physicians to another group of physicians using a deep learning program assistant to improve their diagnoses. Of those studies, 11 stated that the physicians’ abilities to detect fractures were improved when using an AI assistant (20, 25-28, 32, 33, 37, 41, 44, 46). The final method of comparison, used by 8 articles, differed vastly from the other studies. The AI program was fed images of fractures that had already been diagnosed by practicing physicians. This diagnosis was referred to as the “ground truth”. The AI would then make a diagnosis that was then directly compared to the ground truth to see how they differed (18, 19, 34, 35, 38, 39, 47, 49). These articles showed the AIs’ varying levels of sensitivity and specificity in fracture detection when contrasted against the physicians’ initial diagnoses. A summary of the articles included in this review is reported in Table 2, which can be found in the additional information section.
Discussion
As physician workloads constantly increase, so does fatigue, mistakes, and avoidable harm to patients. AI assistance can help physicians reduce diagnostic errors and the time it takes to diagnose in critical situations. Many different machine learning (ML) and advanced deep learning (DL) models exist that can be fine tuned to almost any unique scenario to help physicians diagnose simple fractures quickly, as well as avoid missing complex or occult fractures. This review has identified many variables affecting diagnostics originating from the physician experience, AI model and/or training, fracture location and type, demographic features, and complexity of the diagnosis (fracture, non-fracture, localization, specific classification of fracture).
Specialized Expertise, Training Influence, and Interdisciplinary Collaboration
The reviewed literature indicates variable performance dynamics, with AI demonstrating superiority in their respective areas of focus, comparable effectiveness in the majority of studies, and a suggestion of potential heightened accuracy when assisting physicians in fracture detection. A majority of the studies analyzed diagnostic ability among different physician specialties and/or experience, by comparing accuracy to the AI models. Some studies also compared AI models’ predictive ability against other AI models: one showing the AI model SmartUrgence and BoneView being superior to Rayvolve in the categories of accuracy and specificity (18), another demonstrating that Inception V3 performed better than Resnet-50 in sensitivity and specificity (35). This review found that a majority of AI models performed similarly to experienced specialized physicians and radiologists, and better than less experienced physicians and radiologists. (20-22, 26-29, 33, 36-38, 40, 42-44, 50, 52) Some authors also found that when assisted by AI models, physicians demonstrate an increase in accuracy, sensitivity, specificity (20, 22, 25-28, 32, 33, 37, 44, 46), and reduction in time reading images (20, 24, 41, 46). Contrarily, one study found that orthopedic surgeons performed better than the AI model in detecting visually present and occult scaphoid fractures on XRay alone (48). Another significant factor we identified in this review was the quality of model training. Typically, with most variables controlled, all models performed much better when provided more training images. This positive correlation allows the AI models to perform on par with the advanced specialties, but it also goes in the reverse. One AI model performed on par with inexperienced physicians, rather than better, due to a severely small training set (17). The reasons this would be acceptable/necessary are the amount of time allotted, imaging availability, and expertise in tuning the AI model.
Anatomical Variations and Methodological Considerations
In examining the findings from studies that focused on specific anatomical regions, many shed light on the inherent limitations associated with fracture detection in certain anatomical structures. Cohen et al, focusing on wrist fractures, highlighted the variability in AI performance across different anatomical areas, revealing challenges in detecting carpal bone fractures, especially when excluding the scaphoid bone. The study suggested that the AI algorithm’s training, primarily based on radiographs rather than incorporating clinical information, might contribute to limitations in sensitivity (56% sensitivity for carpal bones as opposed to 83% for scaphoid fractures) (22). Gaps in knowledge were highlighted, where Lindsey et al’s reliance on radiographs alone, without clinical context, might have led to potential misdiagnoses and inflated sensitivity (33). Rather than relying on ground truths set by orthopedic surgeons using only radiographs, others established ground truth with radiologists incorporating clinical data and follow-up examinations, capturing a more comprehensive spectrum of complex carpal fractures (22, 33).
Kim et al delved into the intricacies of foot fractures, acknowledging the complexities arising from the inherent deformities of the human foot, such as hallux valgus. Despite the success of the AI model, the study recognized instances of misinterpretations, particularly in stress fractures involving repetitive force and cases with severely bent or deformed feet and overlapping bones (46). The unique characteristics of pediatric bone development, such as physeal fractures, less bone density, higher cartilaginous content, and thinner soft tissues, have influenced the complexity of fracture detection (39). With nine of the studies in this scoping review utilizing a pediatric patient population, they collectively underscore the importance of considering anatomical variations and deformities when assessing the diagnostic performance of AI in fracture detection. These findings highlight the need for ongoing refinement and awareness of potential limitations in diverse clinical scenarios.
Synergistic Advancements: AI-Assisted Physician Fracture Detection
The scoping review also revealed a distinct approach that some papers took, where physicians were compared in their fracture detection capabilities when also assisted by a deep learning program. The results consistently showed an improvement in fracture detection skills among physicians, spanning from less experienced interns to seasoned orthopedic attendings and fellows, with the aid of an AI program. For example, Kim et al investigated their AI model’s (Score-CAM) effectiveness using two testing sets, where participants diagnosed raw radiographs and labeled radiographs with overlaid heat maps indicating foot fractures. Aside from the study revealing a strong agreement between expert diagnoses and AI interpretations, it also resulted in a substantial overall accuracy improvement, increased diagnostic rate (radiographs per minute), and reduced diagnosis time (46). Other studies in agreement also indicated AI’s potential for both training and assisting clinicians, especially those with less experience (25-28). This reveals an interesting integration, where AI can provide valuable support across all levels of expertise, fostering a synergistic relationship that optimizes patient care and outcomes.
Implications for Future Research
To address potential bias and improve the generalizability of findings, future studies should incorporate a more diverse and representative sample of physicians. Moreover, there is a critical need to explore and articulate the features learned by deep learning algorithms, enhancing transparency and interpretability in AI-assisted diagnosis. Considering the exclusive focus on distinguishing between healthy and fractured bones, future research should expand the scope to include a broader range of pathological presentations, fostering a more comprehensive understanding of AI’s diagnostic capabilities. Additionally, investigations into the integration of AI algorithms into routine clinical pathways, considering real-world challenges, and refining models for diverse anatomical scenarios would contribute to the practical applicability of these technologies in healthcare settings.
Limitations
One article even showed the AI outperform 3 hand surgeons in diagnosing distal radius fractures, but it was limited in that the physicians did not have clinical background information, and the AI was structured to simply determine if there was a fracture present but could not localize the lesion (31). These images were cropped in a way that made it difficult to diagnose for physicians, and they were not given information such as location of hand pain.
The reviews examining the comparison between AI and physicians in diagnosing appendicular skeletal fractures highlighted several common limitations. Firstly, there’s a risk of bias in the way the system is defined as an AI tool, potentially influencing the study outcomes. Moreover, the studies often had a limited number of participating physicians, making it challenging to generalize the findings to a broader medical context. Another shared challenge is the difficulty in understanding the features learned by the deep learning algorithms, leading to uncertainty about the exact elements used for identifying fractures. This leaves a concern about the selective bias introduced by algorithms trained specifically for distinguishing between healthy bones and fractures, potentially overlooking other medical conditions.
Furthermore, the reliance on certain types of radiographic views, such as oblique views not routinely used in hospitals, presents a practical limitation in some studies. This choice, combined with algorithms focusing solely on discriminating between healthy and fractured bones, may miss other abnormalities. Integrating these algorithms into real-world clinical pathways also poses a challenge, requiring further studies to validate their impact. The studies commonly noted the limited generalizability of some models, trained exclusively at a single institution, and potential inaccuracies due to false-positive cases related to poor film positioning and artifacts, as well as false-negative cases involving non-displaced fractures. These shared limitations emphasize the need for careful consideration and ongoing refinement in the development and application of AI systems in fracture diagnosis.
Conclusion
This review demonstrates that the future of medicine may soon be able to combine the use of Artificial Intelligence in diagnostics. Almost all of the recent research on AI in fracture diagnosis shows that physicians could benefit with the implementation of AI in some part of their work processes. AI consistently performs better than most inexperienced physicians and comparably to specialists. This indicates that anyone from new residents to moderately experienced radiologists, can benefit from AI that shows similar performance to seasoned experts. Missed or delayed diagnosis of fractures are often a litigation concern for hospitals, but more so can impact patient healing and morbidity depending on the fracture type. Deep learning models have proven that when trained properly, they can reduce physician reading times, missed diagnoses, over ordering of advanced imaging, and falsely diagnosing a fracture when none exists. These benefits can be seen anywhere, but are also especially significant in the cases of facilities with smaller staff of specialists and less access to advanced imaging. While limitations are still present in current AI models across the library of research, the future in which most of these concerns are solved is looking bright.
Additional Information
Table 2 provides a comprehensive summary of the included articles, highlighting their methods, key findings, and noted limitations to offer a clear overview of the evidence base.
Conflict of Interest
The authors of this scoping review can attest this content is free from all conflicts.
References
- Garraway WM, Stauffer RN, Kurland LT, O’Fallon WM. Limb fractures in a defined population. I. Frequency and distribution. Mayo Clin Proc. 1979;54(11):701-707.
- Adler CP. Pathologische Knochenfrakturen: Definition und Klassifikation [Pathologic bone fractures: definition and classification]. Langenbecks Arch Chir Suppl II Verh Dtsch Ges Chir. 1989;479-486.
- Melton LJ. Chapter 1 – Epidemiology of Fractures. ScienceDirect. January 1, 1999. https://www.sciencedirect.com/science/article/abs/pii/B9780125286404500022
- Kanis JA. Assessment of fracture risk and its application to screening for postmenopausal osteoporosis: synopsis of a WHO report. WHO Study Group. Osteoporos Int. 1994;4(6):368-381. doi:10.1007/BF01622200
- Kelsey JL, Samelson EJ. Variation in risk factors for fractures at different sites. Curr Osteoporos Rep. 2009;7(4):127-133. doi:10.1007/s11914-009-0022-3
- Fritz B, Yi PH, Kijowski R, Fritz J. Radiomics and Deep Learning for Disease Detection in Musculoskeletal Radiology: An Overview of Novel MRI- and CT-Based Approaches. Invest Radiol. 2023;58(1):3-13. doi:10.1097/RLI.0000000000000907
- Razavian N, Knoll F, Geras KJ. Artificial Intelligence Explained for Nonexperts. Semin Musculoskelet Radiol. 2020;24(1):3-11. doi:10.1055/s-0039-3401041
- Guermazi A, Tannoury C, Kompel AJ, et al. Improving Radiographic Fracture Recognition Performance and Efficiency Using Artificial Intelligence. Radiology. 2022;302(3):627-636. doi:10.1148/radiol.210937
- Tobler P, Cyriac J, Kovacs BK, et al. AI-based detection and classification of distal radius fractures using low-effort data labeling: evaluation of applicability and effect of training set size. Eur Radiol. 2021;31(9):6816-6824. doi:10.1007/s00330-021-07811-2
- Raisuddin AM, Vaattovaara E, Nevalainen M, et al. Critical evaluation of deep neural networks for wrist fracture detection. Sci Rep. 2021;11(1):6006. Published 2021 Mar 16. doi:10.1038/s41598-021-85570-2
- Kim DH, MacKinnon T. Artificial intelligence in fracture detection: transfer learning from deep convolutional neural networks. Clin Radiol. 2018;73(5):439-445. doi:10.1016/j.crad.2017.11.015
- Cheng CT, Ho TY, Lee TY, et al. Application of a deep learning algorithm for detection and visualization of hip fractures on plain pelvic radiographs. Eur Radiol. 2019;29(10):5469-5477. doi:10.1007/s00330-019-06167-y
- Cheng CT, Wang Y, Chen HW, et al. A scalable physician-level deep learning algorithm detects universal trauma on pelvic radiographs. Nat Commun. 2021;12(1):1066. Published 2021 Feb 16. doi:10.1038/s41467-021-21311-3
- Nich C, Behr J, Crenn V, Normand N, Mouchère H, d’Assignies G. Applications of artificial intelligence and machine learning for the hip and knee surgeon: current state and implications for the future. Int Orthop. 2022;46(5):937-944. doi:10.1007/s00264-022-05346-9
- Hornung AL, Hornung CM, Mallow GM, et al. Artificial intelligence in spine care: current applications and future utility. Eur Spine J. 2022;31(8):2057-2081. doi:10.1007/s00586-022-07176-0
- Murata K, Endo K, Aihara T, et al. Artificial intelligence for the detection of vertebral fractures on plain spinal radiography. Sci Rep. 2020;10(1):20031. Published 2020 Nov
- Blüthgen C, Becker AS, de Martini IV, Meier A, Martini K, Frauenfelder T. (2020). “Detection and localization of distal radius fractures: Deep learning system versus radiologists.” European Journal of Radiology 126.
- Bousson V, Attané G, Benoist N, et al. (2023). “Artificial Intelligence for Detecting Acute Fractures in Patients Admitted to an Emergency Department: Real-Life Performance of Three Commercial Algorithms.” Academic Radiology.
- Bulstra, A. E. J. and C. Machine Learning (2022). “A Machine Learning Algorithm to Estimate the Probability of a True Scaphoid Fracture After Wrist Trauma.” Journal of Hand Surgery-American Volume 47(8): E14-718.
- Canoni-Meynet L, Verdot P, Danner A, Calame P, Aubry S. (2022). “Added value of an artificial intelligence solution for fracture detection in the radiologist’s daily trauma emergencies workflow.” Diagnostic and Interventional Imaging 103(12): 594-600.
- Choi JW, Cho YJ, Lee S, et al. (2020). “Using a Dual-Input Convolutional Neural Network for Automated Detection of Pediatric Supracondylar Fracture on Conventional Radiography.” Investigative Radiology 55(2): 101-110.
- Cohen M, Puntonet J, Sanchez J, et al. (2023). “Artificial intelligence vs. radiologist: accuracy of wrist fracture detection on radiographs.” European Radiology 33(6): 3974-3983.
- Gipson J, Tang V, Seah J, et al. (2022). “Diagnostic accuracy of a commercially available deep-learning algorithm in supine chest radiographs following trauma.” British Journal of Radiology 95(1134).
- Hendrix N, Hendrix W, Dijke KV, et al. (2023). “Musculoskeletal radiologist-level performance by using deep learning for detection of scaphoid fractures on conventional multi-view radiographs of hand and wrist.” European Radiology 33(3): 1575-1588.
- Hrzic F, Tschauner S, Sorantin E, Štajduhar I. (2022). “Fracture Recognition in Paediatric Wrist Radiographs: An Object Detection Approach.” Mathematics 10(16): 23.
- Lee KC, Choi IC, Kang CH, et al. (2023). “Clinical Validation of an Artificial Intelligence Model for Detecting Distal Radius, Ulnar Styloid, and Scaphoid Fractures on Conventional Wrist Radiographs.” Diagnostics 13(9).
- Nguyen T, Maarek R, Hermann AL, et al. (2022). “Assessment of an Artificial Intelligence aid in detection of pediatric appendicular skeletal fractures by senior and junior radiologists.” Insights into Imaging 14: 36-37.
- Mawatari T, Hayashida Y, Katsuragawa S, et al. (2020). “The effect of deep convolutional neural networks on radiologists’ performance in the detection of hip fractures on digital pelvic radiographs.” European Journal of Radiology 130: 7.
- Oakden-Rayner L, Gale W, Bonham TA, et al. (2022). “Validation and algorithmic audit of a deep learning system for the detection of proximal femoral fractures in patients in the emergency department: a diagnostic accuracy study.” The Lancet Digital Health 4(5): e351-e358.
- Anttila TT, Karjalainen TV, Mäkelä TO, et al. (2023). “Detecting Distal Radius Fractures Using a Segmentation-Based Deep Learning Model.” Journal of Digital Imaging 36(2): 679-687.
- Suzuki T, Maki S, Yamazaki T, et al. (2022). “Detecting Distal Radial Fractures from Wrist Radiographs Using a Deep Convolutional Neural Network with an Accuracy Comparable to Hand Orthopedic Surgeons.” Journal of Digital Imaging 35(1): 39-46.
- Tanzi L, Vezzetti E, Moreno R, Aprato A, Audisio A, Massè A. (2020). “Hierarchical fracture classification of proximal femur X-Ray images using a multistage Deep Learning approach.” European Journal of Radiology 133: 109373.
- Lindsey R, Daluiski A, Chopra S, et al. (2018). “Deep neural network improves fracture detection by clinicians.” Proceedings of the National Academy of Sciences of the United States of America 115(45): 11591-11596.
- Aryasomayajula S, Hing CB, Siebachmeyer M, et al. (2023). “Developing an artificial intelligence diagnostic tool for pediatric distal radius fractures, a proof of concept study.” Annals of the Royal College of Surgeons of England.
- Ashkani-Esfahani S, Yazdi RM, Bhimani R, et al. (2022). “Detection of ankle fractures using deep learning algorithms.” Foot and Ankle Surgery 28(8): 1259-1265.
- Cheng CT, Wang Y, Chen HW, et al. (2021). “A scalable physician-level deep learning algorithm detects universal trauma on pelvic radiographs.” Nature communications 12(1): 1066.
- Cheng CT, Chen CC, Cheng FJ, et al. (2020). “A Human-Algorithm Integration System for Hip Fracture Detection on Plain Radiography: System Development and Validation Study.” Jmir Medical Informatics 8(11): 13.
- Cheng CT, Ho TY, Lee TY, et al. (2019). “Application of a deep learning algorithm for detection and visualization of hip fractures on plain pelvic radiographs.” European Radiology 29(10): 5469-5477.
- Cheng CT, Hsu CP, Ooyang CH, et al. (2023). “Evaluation of ensemble strategy on the development of multiple view ankle fracture detection algorithm.” British Journal of Radiology 96(1145).
- Chung SW, Han SS, Lee JW, et al. (2018). “Automated detection and classification of the proximal humerus fracture by using deep learning algorithm.” Acta Orthopaedica 89(4): 468-473.
- Duron L, Ducarouge A, Gillibert A, et al. (2021). “Assessment of an AI Aid in Detection of Adult Appendicular Skeletal Fractures by Emergency Physicians and Radiologists: A Multicenter Cross-sectional Diagnostic Study.” Radiology 300(1): 120-129.
- Gan K, Xu D, Lin Y, et al. (2019). “Artificial intelligence detection of distal radius fractures: a comparison between the convolutional neural network and professional assessments.” Acta Orthopaedica 90(4): 394-400.
- Gasmi I, Calinghen A, Parienti JJ, Belloy F, Fohlen A, Pelage JP. (2023). “Comparison of diagnostic performance of a deep learning algorithm, emergency physicians, junior radiologists and senior radiologists in the detection of appendicular fractures in children.” Pediatric Radiology 53(8): 1675-1684.
- Guermazi A, Tannoury C, Kompel AJ, et al. (2022). “Improving Radiographic Fracture Recognition Performance and Efficiency Using Artificial Intelligence.” Radiology 302(3): 627-636.
- Hayashi D, Kompel AJ, Ventre J, et al. (2022). “Automated detection of acute appendicular skeletal fractures in pediatric patients using deep learning.” Skeletal Radiology 51(11): 2129-2139.
- Kim T, Goh TS, Lee JS, Lee JH, Kim H, Jung ID. (2023). “Transfer learning-based ensemble convolutional neural network for accelerated diagnosis of foot fractures.” Physical and Engineering Sciences in Medicine 46(1): 265-277.
- Kim T, Moon NH, Goh TS, Jung ID. (2023). “Detection of incomplete atypical femoral fracture on anteroposterior radiographs via explainable artificial intelligence.” Scientific reports 13(1): 10415.
- Langerhuizen DWG, Bulstra AEJ, Janssen SJ, et al. (2020). “Is Deep Learning on Par with Human Observers for Detection of Radiographically Visible and Occult Fractures of the Scaphoid?” Clinical Orthopaedics and Related Research 478(11): 2653-2659.
- Lind A, Akbarian E, Olsson S, et al. (2021). “Artificial intelligence for the classification of fractures around the knee in adults according to the 2018 AO/OTA classification system.” PLoS ONE 16(4).
- Murphy EA, Ehrhardt B, Gregson CL, et al. (2022). “Machine learning outperforms clinical experts in classification of hip fractures.” Scientific reports 12(1): 2058.
- Ozkaya E, Topal FE, Bulut T, Gursoy M, Ozuysal M, Karakaya Z. (2022). “Evaluation of an artificial intelligence system for diagnosing scaphoid fracture on direct radiography.” European journal of trauma and emergency surgery : official publication of the European Trauma Society 48(1): 585-592.
- Twinprai N, Boonrod A, Boonrod A, et al. (2022). “Artificial intelligence (AI) vs. human in hip fracture detection.” Heliyon 8(11): 8.